Discover the Reality of
Scientific Mythology
The Facts of Self-Animating Networks in Nature and a New, Realistic Role for the Mythic Imagination
|
|
> NOTICE: This website represents a work-in-progress. Please contribute your feedback! email link <
Home Page
| Netology: Understanding the Logic of Network Dynamics
|
|
Different Network Structures Create Radically Different Network Dynamics
Bottom Line:
1. All things and events manifest as networks:
From water molecules to waterfalls and persons to social relationships,
the parts and actions that create these are systems which can be represented as network
structures.
2. Different types of network structure create different effects:
Network structure can create orderly, predictable effects or more
complex and unpredictable effects. Most order in Nature is created by network
structure which manifests unpredictable, autonomously self-organizing behavior. This network
autonomy is pervasive, beyond our control, and effectively animates both our selves and the biosphere.
3. Understanding the effects of network autonomy is essential knowledge of reality: Distinguishing types of network structures and their effects reveals how the world actually works. To
think like Nature acts, and act accordingly, requires perceiving the
mysterious creativity of network autonomy in and all around us.
|
|
> Summary Overview <
Networks are everywhere -- But They are not all the same
The concept of networks is used to describe the ways parts or factors
are connected and influence each other to create things, events, even
ideas. Network modeling assists in understanding how things exist and
function as set of relationships among connected parts that for a
systesm. But different types of network structure associate with very
different effects.
Networks are relationships of parts
Four lines in a particular network structure constitute a square:
Different types of Networks have different Dynamical Effects
Representing events and systems as networks allows us to identify
relationships between parts and how these influence each other.
Systems of parts that can change configuration, thus their properties
and effects, are manifest dynamic networks. Simple mechanical networks
are generally predictable in the changes these can produce. But the
connections and influences of complex
networks can "do" things that simple, mechanical networks
cannot do.
Dynamic Networks can change system behavior
A basic electrical
circuit network:
A complex social network:
Less
complex system networks manifest more consistent, predictable behavior.
More complex ones create interdependent actions in each moment and
recursive feedback loops that cannot be fully identified and sequence.
Networks are bi-dynamical
Progressively
consistent
dynamics :
Inconsistent, interdependent dynamics:
As in 1,2,3 . . .
As in 1,3,5, . . .
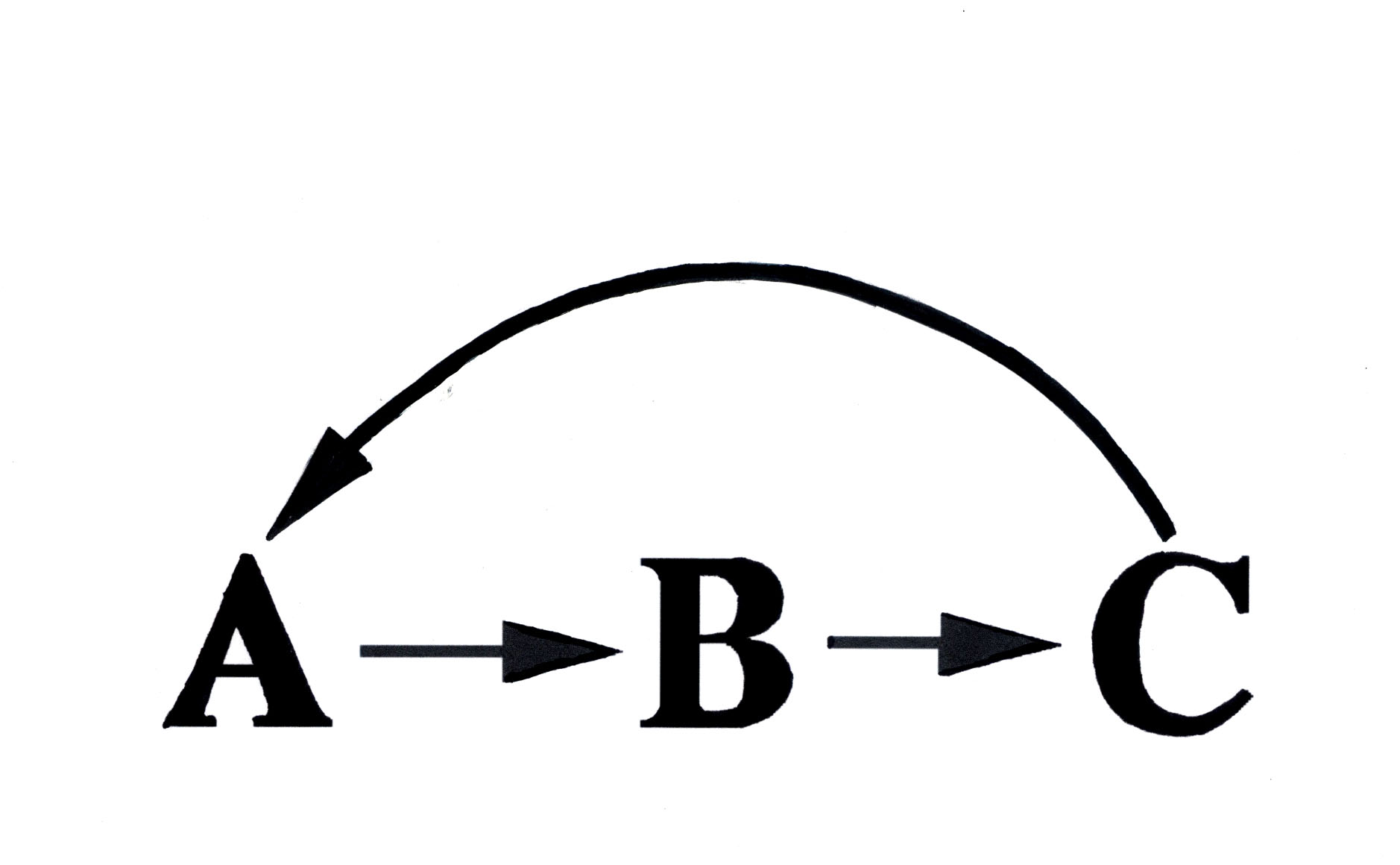
Network Structure Makes a Difference
Simpler net
structure
More complex net structure
Simpler networks describe predictably mechanical relationships. But greater
diversity and connectivity of parts in a system can lead to levels of
interdependency that generate complexity's synergistic dynamics. This
can produce unpredictable system properties, such as autonomously
self-organizing network operations. Recent science has
revealed that most of the forms and functions in the biosphere are
generated by complex autonomous networks--not the hierarchically
structured ones of predictably mechanistic dynamics. We need to learn
to see our selves and the world as complex networks if we want to
realistically understand how the world works. The
trouble is, these networks are effecitively invisible. We can model
their structure and calculate some of their dynamics, but not all.
Networks autonomously manifest Archetypal Dynamical Character
The emergent origins of complex adaptive networks gives them
autonomous intentionality. That enables them to act in ways that both
sustain and adapt the operations of their systems. This self-organizing
capacity is unpredictable, yet also expresses patterns of behavior
that identify types of systems, such as plants, humans, or economies.
These differentiating traits are archetypal, indicating characteristic
patterns and behaviors that tend to arise from types of system parts
networked in certain ways. But each manifestation of a type of network
is also individualized by its network autonomy--no two are identical
even when very similarly configured.
Thus every network is a particularized manifestation of its
background archetypal character. To understand each is to investigate
how it individualizes its archetypal origins as its unique,
unpredictable yet intentional network autonomy. Network science assists
in characterizing the archetypal traits of network behavior in terms of
dynamical attractors.
Network behavior has dynamical character
Predictable fixed point and periodic attractor patterns versus
an unpredictable yet self-ordering strange attractor:
Seeing Networks
Representing things and events as networks requires
identifying
the parts of a given system and how these effect each other. This
analysis provides a way of illustrating how any given thing or event is
the
manifestation of those relationships, whether the form of a building or
the behavior of a person. To "see" networks is to "see"
relationships between connected parts and actions. These relationships
are not the same as a thing itself. The emergent origins and operations of complex networks are effectively hidden from direct observation. We can only directly access part of the
system dynamics that manifest them. Thus abstract modeling is required
to create visualize networks as types of relationships among system
parts.
To "see" networks is to model relationships
Network dynamics are a partly invisible aspect of a system's operations:
Thus seeing networks involves
perceiving something that is
typically hidden by the literal appearances of things and events. That
requires a sort of "x ray" vision that "abstracts" the relationships
from the
whole which these create, in order to perceive the manner of its being
what
it is. The more dynamic and interactive networks become, the more
complex are the relationships that generate them, and thus the more
difficult they are to perceive.
How network science sees the relationships of complex networks
Simpler network structures interact interdependently to generate emergent network autonomy:
How
we perceive networks profoundly shapes
our worldview. But our present modern mentality is so obsessed with
control we reflexively perceive networks as hierarchies of sequential
relationships, since this view suggests we can manipulate and manage
them. Thus we are inherently resistant to perceiving concurrently
interdependent relationships, with the network
autonomy these produce, since that perspective reveals a Nature we
cannot control. Worse yet,
the new science shows that our attempts to control our environments is
crippling the self-sustaining autonomy of natural systems.
How we look is what we see
Seeing networks as hierarchical versus interdependent sets of relationships:
Complex Networks act Autonomously to Animate the World
Complex networks emerge from
partly disorderly relationships among interdependent system parts
through a dynamical condition termed self-organizing criticality. It is
this uncertain interactivity that appears to provide them with the
capacity to process information and effectively make decisions about
how their system will organize its operations--either by repeating past
actions or rearranging itself to operate in new ways that could prove
more adaptively sustainable. Thus network autonomy effectively create and animates a system.
This autonomous, intentionally
self-organizing influence acts in response to changing conditions
within a system and in its external environments. Many system networks
interacting with each other manifest larger scales of autonomous
networks, such as societies and ecologies. In so doing, complex
networks act reciprocally to generate most of the order and functions
in the biosphere around us through their interdependent autonomy--they
literally animate the world.
The emergent network nature of Nature
Reciprocal interactions among many self-animating networks manifests
larger self-organizing networks, such as ecologies:
Understanding how complex networks make us and the world is essential
to thinking like Nature acts, thus to our very survival. But this shift in our thinking requires a radical change in how we understand the origins of order in and around us. We require a new, "netological" philosophy.
To Know More Dive Down |
Contents Below with Links
Network Dynamics and Their Radical Implications
Bi-Dynamical Order Creation and Complexity's Autonomous Networks
Perceiving the different ways network
relationships create order is essential to understanding the autonomous
self-organization of natural systems. For that we need the insights of
complexity and network science. The implications of those insights,
explored below, can be summarized as follows:
1. Bi-Dynamical Order Creation:
Order is created through two types of dynamical phenomena. The first is
composed of sequentially dependent events that are fully
identifiable and predictably deterministic--as described in the Laws of
Physics. The second presumably arises from the first, but is composed
of simultaneously interdependent events that are not fully identifiable
and are unpredictably deterministic--as described in the science of
chaos and complexity.
The first type is proportionally consistent, thus fully explainable and
can be characterized as mechanistic. The second type is
disproportionally creative, thus not fully explainable, and so is
termed emergent to distinguish if from the predictable dependency of
mechanistic order creation. Both
modes are involved in the creation of the majority of forms in our
biosphere. Thus it arises from and is sustained by a bi-dynamical
domain of order creation: the mechanistic and the emergent dynamics of order creation. If we are to think like Nature acts, we must think bi-dynamically.
2. Mechanistic versus Emergent System Networks:
Things formed by multiple parts constitute systems. The arrangement of
connections between and activity among those parts constitute a
system's network. Networks can be relatively static or dynamic. A
dinner plate has a more static network of relationships between its
physical parts. A city system has more dynamic network relationships.
Network dynamics can create a system's form and operations through
predictably mechanical events, as in a clock, or through unpredictably
emergent dynamics, as in a city. Unlike mechanical system
networks, the emergent creativity of the networks of complex systems
cannot be fully described or explained. To think like Nature acts we
must think in terms of both types of systems and networks.
4. Network Autonomy:
Emergent networks can produce autonomously self-organizing behavior
that both maintains and adapts the operations of their systems.
Emergent networks can process information and effectively "think for
themselves." Network
autonomy is what makes us alive by intelligently animating our
body/mind systems. But it is also active in non-biological systems like
ecologies and economies. To think the dynamical reality
of most human and non-humans systems we must perceive the pervasive
operations of network autonomy.
5. Collective Network Autonomy:
Multiple autonomous networks interact interdependently to generate collectively
emergent meta-networks, from bodies to economies, ant colonies and the biosphere itself. Most
order in and around us is an emergent property of reciprocally
interdependent autonomous networks.
To perceive the interdependent dynamics of meta-systems such as
ecologies and societies, we must think in terms of radical reciprocity.
6. Archetypal Network Autonomy:
Autonomously self-organizing networks manifest unpredictably variable
yet identifiably characteristic patterns of behavior. Such networks
are partly unique expressions of a characterizing set of qualities that
identify them as types of networks. Rodents constitute an range of
animal traits that identify them as a type of complex system whose
emergent networks express characteristic traits of form and behavior.
These traits are termed archetypal because they are a potential field
of possibilities for being a rodent but do not dictate the exact form
and network behavior of any given rodent system. Mice are a identified
by subset of archetypal traits associated with the characteristic of
mouse network autonomy. A particular mouse is an instance of the
network type of its species. But each individual mouse expresses its
archetypal potential with some uniqueness of network autonomy.At each
level, systems and networks manifest autonomy as archetypally distinct
behavioral personality: rodents, mice, a mouse. To think about how network autonomy creates the world we must think archetypally.
7. Limitations of Autonomous Network Definition:
Emergent
order creation is not fully identifiable through
reductive scientific analysis, thus not completely explainable.
Emergent order creation and network autonomy are effectively invisible.
Our ability to precisely define and understand these aspects of reality
is severely limited, despite the fact that they create the majority of order in the biosphere. To think like Nature acts we must think in more than scientifically reductive ways.
8. Network Autonomy Makes Nature Uncontrollable:
Due to its emergent basis, network autonomy cannot be directly manipulated and controlled. Attempts
to impose external control on autonomous networks can debilitate the
sustainable and adaptive traits of their systems resulting in system collapse. To
live sustainably within the reciprocal network autonomies of the
biosphere we must facilitate rather than disrupt these relationships.
9. Network Autonomy and the Necessity of Intuitive Understanding:
Adequately realistic awareness of how the world works requires some
method of perceiving and conceiving how emergent creativity and network
autonomy "make the world." Because these are deterministic, even
intentional, yet not predictable, knowledge of "how they tend to
behave" and thus how to interact adaptively with them cannot be
precise. But we can intuitively assessing their behavioral tendencies
as sets of archetypal characteristics that constitute the personalities
of autonomy in different networks. Available scientific evidence
provides a basis from which to extend understanding complex systems and
their emergent networks by this intuitively exploring the behavioral
character of these effectively invisible but crucial aspects of reality.
10. Civilization's Aversion to Network Autonomy:
Human
obsession with controlling phenomena leads to creation of hierarchical,
technologically enhanced, control oriented human systems--such as
governments, armies, social institutions, industries, or corporations.
These
reinforce assumptions that all phenomena can and should be controlled.
The resulting human mentality is profoundly resistant to acknowledging
and acting reciprocally with the autonomy of the natural networks that
create and sustain the biosphere. Our debilitating impacts on the
autonomous operations of those networks is causing their collapse.
Our own survival requires us to develop intuitive methods of
understanding the crucial roles of emergence and network autonomy. To
think like Nature acts, and act accordingly, demands a cultural
practice of continually countering our control oriented focus on
mechanistic order creation. The science of complexity and network
autonomy now provide an empirical basis for this cultural
transformation.
The Logic of Network Structure and Dynamics
Networks as Static and Dynamic Relationships among System Parts
The relationships between a system's components are what form
their networks. Whether the system is boat or a human social group, it
is the qualities of the relationships between those parts, how they act
upon each other, that generates the system's recognizable order and
patterns of behavior These relationships can be relatively static,
meaning little change over time, or actively dynamic, meaning there is
ongoing change in how parts are connected and effect each other. The
pattern of more static relationships, like the structure of a molecule
or house, is mostly consistent, thus easy to describe and explain. But
the pattern of complex dynamical relationships, such as the
interactivity among millions of neurons in a human brain, is constantly
variable and virtually impossible to completely describe.
Houses built from the same materials have vastly different forms
and functions because the network of relationships between their parts
varies. But the network relationships of any given house remain mostly
static. The systems of human brains have are based upon similar
materials and a shared general physical structure. But the network of
relationships between their parts is both vastly more complex and
dynamic than that of a boat or house. But in both cases, the network of
relationships constituting a system's network is not a thing in itself.
Thus the network, as a set of relationships between parts, is not
overtly obvious or visible. We can perceive the whole of the system but
often not the traits of all the relationships between its parts
The more complex the dynamics of a system, the more interdependency of
effects its parts have on each other, the more inaccessible to direct
examination become the relationships that constitute its network
operations.
Gaining understanding of
the properties of networks, and the radical differences between those with relatively static or consistent
relationships and those with more dynamically complex sets of
relationships, is aided by abstractly visualizing the traits of how system structure generates them.
Network Structure Topology: Types of Connection Make a Logically Profound Difference
The
most basic insight of network science it its abstract modeling of
how system parts are connected to create the relationships of their
networks. These
models
give us a way of visualizing networks as an abstract topology. These
diagrams represent the logic of placement of system parts in terms of
their networked relationships. Network models do no look like the
systems they represent. Instead, system parts are represented as nodes,
and the relationships between parts as links between those
nodes, standing for some form of connection between parts. These links
indicate pathways
across which system parts influence each other, creating what is termed
feedback. Feedback can take the form of energy, such as heat or
mechanical force, or the exchange of more abstract information, by way
of which parts influence each other, and thus generate the overall
operations of a network. Feedback is particularly crucial to the
interdependent dynamics of complex systems. It is
characterized as input and output. System parts are effected by the
outputs of other parts that influence them as inputs, then respond
with outputs of their own. So feedback is a flow of inputs and outputs
that passes between system parts and between distinct systems.
The
patterning of connections between nodes influences how inputs and
outputs among system parts aggregate to the overall dynamics and
operation of a system. Networks
can have more linearly
sequenced patterns of linkage between nodes, as in a simple series of
nodes each connected to the next in a line. But connecting links
between nodes can also be or more multiple, creating redundant
patterns of linkage. The latter are represented by the "mesh" and
"fully
connected" examples in the illustration below.
Types of network topologies promote different network properties
Abstract models of different patterns of node linking or connectivity:
Linearly sequenced connections, in
which one node is connected to only one or two others, limit the flow
of feedback between nodes. Actions and reactions of system part, as
inputs and outputs, tend to be more sequentially dependent when linked
in this manner. This type of network connectivity is typical of
mechanical systems like clocks and motors. More
multiply connected patterns, as in mesh and fully connected models, can
amplify the amount and simultaneity of
feedback between nodes. Thus the latter tend to generate more
interdependency of actions and reactions, leading to more of the
nonlinear dynamics of complex systems, with their emergent properties
and self-organizing networks.
In the illustration below are models of a centrally
structured pattern of connections, having a single hub with multiple
links, then a de-centered pattern, having multiple hubs, and lastly a
distributed pattern, in which most nodes are multiply connected to
other nodes. Nodes with multiple links are termed hubs. These often act
as organizing factors in the flow of feedback across a system.
Three basic types of network topology
Centralized single hub:
De-Centralized multi-hub:
Distributed fully connected:
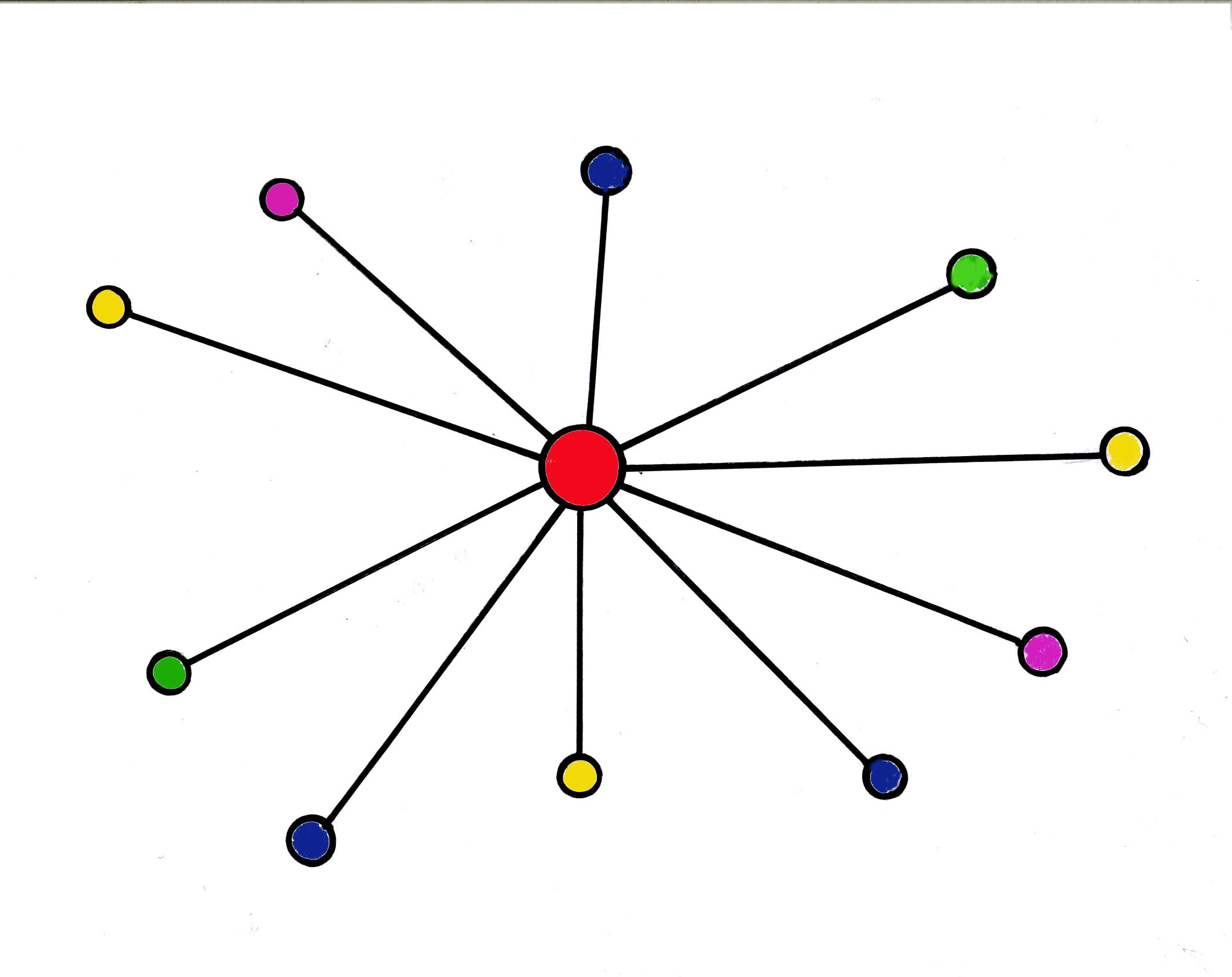 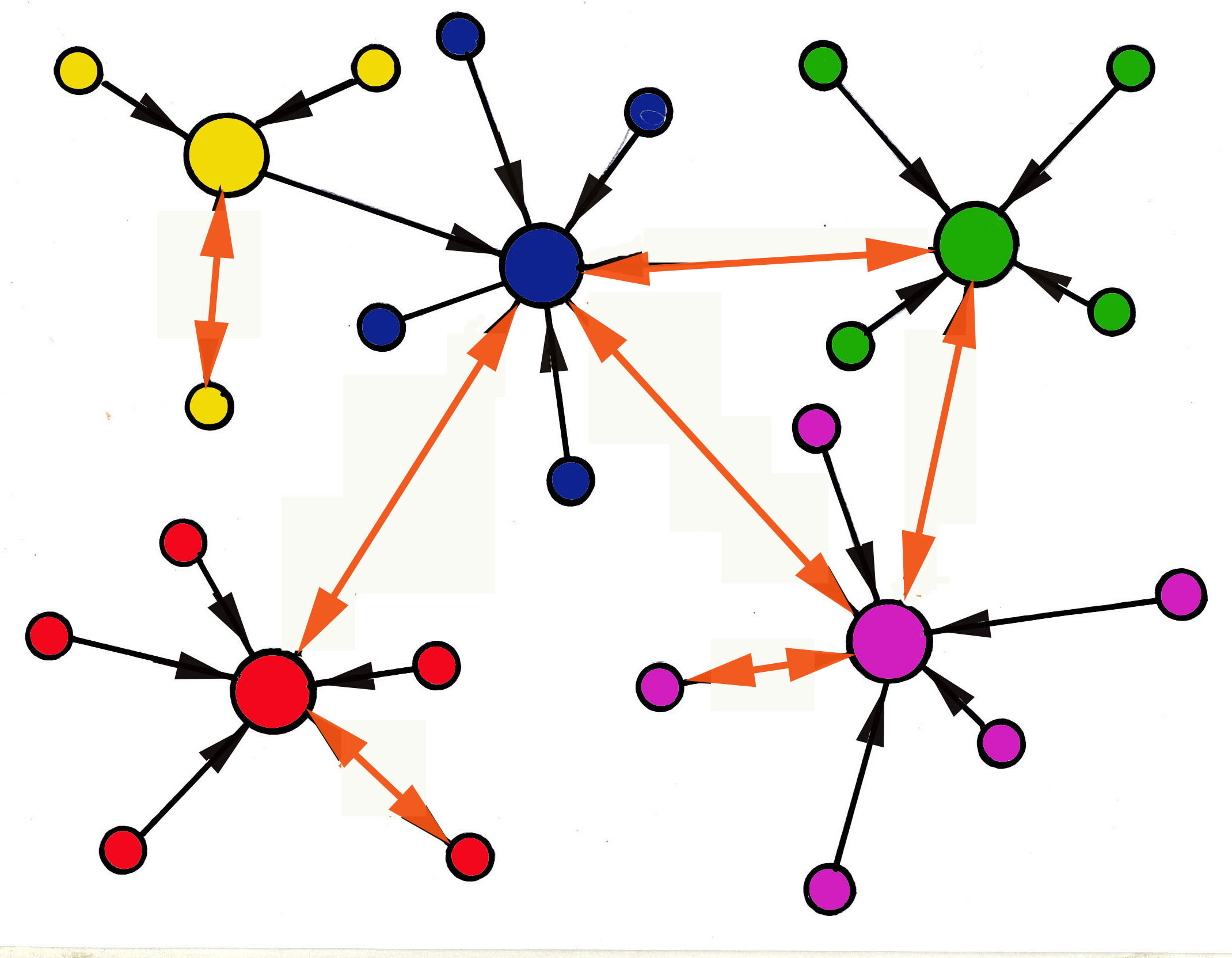
The arrows on the link
lines indicate directions of flow of feedback between nodes. Links can
represent transmission of input and output influence as traveling in
either a single
direction or both in directions between nodes. The orange
arrows pointing in both directions identify links that transmit
feedback in both directions, which can occur simultaneously,
making the resulting effects difficult or impossible to
distinguish. But even single direction flows of feedback can circulate
around loops of node connection, feeding back into itself in ways that
can add additional aspects of input and output. Another mode of representing multiply
connected networks positions the nodes on a circle and draws links across
the circle, giving a further way of imaging these redundant connections
and their simultaneous flows of feedback
Peripherally modeled network topology
Network nodes are placed on a circle with links between
and color coding indicating more closely connected nodes:
This modeling can be further amplified by representing the network more
three-dimensionally, giving us a slightly more real world impression of
network structure..
Even in
these simplistic diagrams it becomes possible to conceive how feedback
between nodes can become mutually modifying, leading to changes in feedback influence across a network. Outputs from two or more
nodes can effect each other simultaneously, creating further factors
that act as outputs and inputs: feedback can change itself as it flows around the
network, creating patterns of simultaneous interdependency that cannot
be effectively sequenced. It is this type of system structure that particularly
associates with emergent properties and network self-organizing
autonomy.
Predictable causes and effects can be understood as
linear sequences of action and reaction in which each is dependent upon
the preceding one. These can aggregate into convergent flows of causes
and effects, resulting in a single specifiable condition.
Predictably deterministic dynamics
Succeeding events, each dependent upon the previous--
differentiable dynamical progression:
But network
interdependency results in an ongoing flow of concurrently
interdependent influences having synergistic effects that do not
manifest this hierarchic
progression: there is no straight line to the causal effects. Thus we
must attempt to think of them as having instantaneous interactions
involving feedback that pass through multiple network nodes then
loops back to prior ones, circling round and round in an
ultimately un-differentiable confusion.
Unpredictably deterministic dynamics
Simultaneously interdependent events--Undifferentiable dynamical interaction:
This thought helps
understand how complex systems and their networks become not only
unpredictably emergent but also cannot be controlled. There is no way
to access their interdependencies directly and manipulate these like
parts of a machine. When we do attempt to manipulate their
interdependent, emergent operations, this interference can cause
catastrophic failure of network self-organization.
The
basic elements of network modeling provide a way of conceptualizing the
differences between forms and events that are predictable and
explainable, and those that are not. When we "see" a house as an
architectural drawing, we conceive it as a non-complex network of
predictably dependent structural relationships, of nodes and links,
that can be fully quantified and calculated. We can explain how such a
physical network functions. But when we "see" house as an expression of
a cultural network, it becomes an effect of a complex adaptive system
with a very different type of network structure--one with dynamic
relationships that are not predictably dependent, thus which we cannot
fully map or explain. But that awareness assists in realizing why the
meaning of a house as a physical structure versus as a cultural
phenomena is profoundly different.
While these models are
useful in conceiving differences in structure, they are only reductive
abstractions, not literal pictures of actual, real world systems and
their networks. They cannot provide us with any adequate sense
of the simultaneously interdependent activity in complex networks or
their continually variable operations. Representing
such network operations in real time, as they happen, is astonishingly
difficult, if it is possible. Complex networks are by definition rarely
static.
Connections between nodes can be modified or created moment to moment,
changing the operational properties of the network and thus
configuration of the system as it responds to changes in its
environment. An extreme version of this dynamical flexibility is the
metamorphic event of a caterpillar's self-induced network
transformation into a butterfly. In this instance a network completely
re-self-organizes its system and its self into radically different
forms and functions. But most natural systems are doing this to some degree on an ongoing basis.
Video graphics assist somewhat in modeling
these types of network
activity and transformation. But even with moving images the challenge
of representing simultaneity is profound.
Though network modeling is useful in getting some logical impression of
how complex networks operate, it is often not possible to fully model
their structures and activities. It is in this regard that
complex networks can be said to be effectively invisible to our
objective, mechanically based perceptions. Further consideration of the
logic of how these networks "do what they do" assists in appreciating
this limitation on our capacity to fully analyze them.
The Logic of Complex Network Dynamics: Emergent Self-Organization from Disorder
The traits of networks associated with
complexity and system-autonomy are in effect anti-structural in
that there is no progressive transition from one single instance of structure to
the next. The structure and activity in one moment can emerge from that
of the preceding moment in ways that are disproportional and
unpredictable, yet are not randomly accidental. The given structure of any
moment does not fully determine that of the next. So successive structural
states of network and system are not entirely dependent upon preceding
ones. These facts underscore the evident relationship between such
uncertainty and the emergence of a network's capacity to change its
operations and modify it systems--though this provides no exact causal
explanation for such phenomena.
It is here that the fundamental question about
emergent causality arises. What can possibly be creating order out of
this discontinuous thus disorderly activity? According to our standard
cultural
definition of causation, changes occur as direct results of preceding
events, as actions that have proportionally consistent thus predictable
effects. You throw a ball with X amount of force and it travels a
predictable distance. But the dynamics of complexity, and particularly
complex adaptive systems, do not manifest this progressive causal
sequencing. Thus one can consider that possibility that an additional
causal condition is generated by complexity's dynamical interdependency
of factors, suggesting a bi-dynamical domain of causal conditions. One
is the familiar domain of the Laws of Physics, that predictably
constrain what can and cannot happen to matter and energy. The other is
the unpredictable but not random order creation of complexity.
Presumably, the latter derives from the former in that certain
relationships among physical entities enables complex dynamics and
thereby emergent causation of otherwise not possible forms organization
and autonomy. But how does this work? What governs this unpredictable
causal phenomena?
Physical science has provided much information
the dynamical conditions involved in this conundrum but not conclusive
description or explanation. But complexity science does provide further
insights.
The property of inconsistent and disproportional emergence associates
with what is termed self-organizing criticality in complex network
activities. Criticality refers to a condition in which a system's
structure is on the verge of significant change in its ordering and
operations. A subsequent shift can either
result from a network re-organizing or
collapsing into more chaotic dynamics that cannot sustain the general
form and operations of the system. Criticality relates to a notion
called the edge of chaos, represented as a boundary zone between the
self-sustaining dynamics of complexity and the more erratic, though
still organization generating ones of chaotic dynamics.
Thus a spectrum is conceived that shows
consistently ordered or stable activity on one end that gives way to
the partly disorderly dynamics of complexity's more radical
interdependency, then the boundary of criticality or edge of chaos
where dynamics become fully chaotic, beyond which is randomness. Chaotic
here means activity that is not random, as it can emergently generate
more ordered patterns out of disorder, but cannot sustain that order as do
complex dynamics. Overall complexity increases toward the edge of chaos.
The dynamical range of order creation
From orderly ordering to
complexity's disorderly self-ordering, then chaos' disorderly but not
self-organizing order creation, and lastly non-ordered randomness:
The zone of complexity is sometimes called the creative domain, as this
is where complex order is created. There is a maintenance aspect to
this creativity as well as an adaptive one. Moment to moment,
complex networks continually re-create the ordering operations of their
system's characteristic forms and functions through self-organizing
criticality: even relative continuity of a system, what is referred to
as homeostasis in body self-regulation, is a continually emergent
phenomenon. Adaptive changes in system configuration and network
operation also emerge from this same dynamical condition of
self-regulating criticality, happening near the boundary of chaotic
dynamics. Both are manifestations of network autonomy and
volitional action initiation.
The dynamical range of new order creation
The disorderly yet self-organizing dynamics of complexity near
the "edge of chaos" is the source of new and adaptive order creation:
Potential for emergent creativity appears to
increase with disorder toward the edge of chaos, suggesting that this
is where self-organizing criticality can be maximized. However, that
potential occurs with the risk that network and system will become
dynamically chaotic and thereby lose the capacity to maintain on-going
self-regulation. Thus either
too much ordered stability or too much chaos can disable self-organizing
network operations.
Here we encounter a very fundamental aspect of the logic of complex
networks: more complex forms of organization derive from significant
instability. Despite our reflexive expectations that order creates
order, network analysis shows us that sudden increases or novel changes
in ordered forms and activities logically involve
instability and discontinuity. A system must be complex and unstable
enough to have the potential to manifest a variety of potential forms or operations,
as well as some non-repeating selective operation that can put those
potentials together in a new way.
This lesson is
familiar in the evolution of human science and technology: significant
innovation is discouraged by strict adherence to established concepts,
standards and designs.Only
with the partly disorderly
diversity of complexity do we get emergent properties in general and
autonomously self-organizing system networks in particular. A
significantly new scientific theory or concept of technological systems
is often not logically consistent with preceding concepts. It is an
emergent conceptual network of evidence and related concepts. But there
is a logic to this emergence in that only an inconsistent,
disproportional break with preceding ones can generate genuine
novelty--a creative leap that can necessarily derives from disruption
of preceding conditions that creates new interdependencies.
Similarly, there is also a logic to the fact that the specific actions
and reactions taking place in the disorderly simultaneity of
interdependency in complex networks operating near the edge of chaos
cannot be fully accessed
and described. If many events are both occurring and modifying each
other simultaneously, then there is no sequence to their causal actions
and reactions. There is no progression to quantify and interpret. This
obscurity is represented by the white box versus
black box model of what can be known in mechanistic terms. A white box
indicates a context of dynamic activity that can be fully accessed and
analyzed. A black box suggests dynamics that remain obscure to all
attempts to directly observe them. Thus we can only observe the before
and after effects of complex system interdependcy and network
self-organization. The initial
inputs to and overall outputs of network operations often can be
quantified and calcuclated, but not the transforming events that
create new
organization and network properties.
The "Black Box" obscurity of network operations in complex systems
The feedback dynamics and information processing in emergent networks
are not accessible to direct observation and identification
The Logic of Network Adaptivity: Four Interacting Factors of Complexity in Autonomous Networks
Further insight into the
traits of emergent networks is provided by identification of four
crucial factors. These are identified as diversity of system parts,
types of connectivity between those parts, interdependency of the
relationships between parts, and degree of adaptive capacity that these
enable. So there is diversity, connectivity, interdependency, and
adaptivity. The adaptive factor is also described as a learning
capacity. A network cannot adapt its system unless it has the
capacity to retain information about the history of the system and its
external environment.
These factors can be imagined as four adjustable
dials, with which the relative degree of each can be increased or
decreased. The relationship between these factors has profound effects
and is part of what networks do when organizing or re-organizing their
systems. In general, the optimal range for these factors is moderate.
Extremes of any factor can decrease dynamical complexity and disable
system/network operations.
The four interacting factors of complex system network adaptivity
Moderate ratios of diversity, connectivity, and
system parts relates to their degree of adaptive complexity:
The level of any or all
factors can also be influenced by changes in a system's external
environment, to which the system's network responds. An example is a social system of humans whose external
environment changes to reduce available resources such as food. The
network of the social group might then adapt by dispersing into smaller
groups that migrate to a different local environment in search of food,
reducing overall connectivity, diversity and interdependency.
Similarly, economic systems produce emergent network re-organization
that can increase or decrease these factors. The crucial point is that robust complex system networks arise from moderate levels of these factors
yet also can act to regulate the relative proportions between them
within their own systems: network autonomy can manipulate the basis of
its own emergence.
The Logical Interplay of Dependent and Interdependent Order Creation
The emergence of unpredictably
deterministic order creation from conditions of interactivity among
predictably deterministic dynamics seems mysterious or magical because
it is not specifiable as a sequential mechanistic process, thus not
completely explainable. We can identify the above discussed logic that
simultaneously interdependent, mutually modifying feed back flows
between system parts that are explainably deterministic seems
appropriate to creating exceptional dynamics that could be
synergistically transformative. Nonetheless, those dynamics, because of
non-sequenceable simultaneity, remain beyond complete identification
and evaluation. However, given the evidence for their existence, we can
proceed to explore the interplay of predictably dependent and
unpredictably interdependent dynamics of order creation--the
bi-dynamical reality of Nature.
From predictable causation to unpredictable emergence
The predictable dynamics
of material physics as the basis for the interactive interdependency of
self-organizing criticality and its emergent ordering that in turn
amplifies emergence:

The Logical Networking of Networks
Modeling networks as discreet sets of nodes and links can make
them seem like distinct, even fixed entities. But the complex systems
and networks that compose Nature are in fact so dynamically active,
variable, and interdependent that their boundaries and relationships
are elusive. Examine any complex system in detail and you are likely to
find it is actually a system of systems and a network of networks.
The Logic of Patchwork Interdependency and Aggregate Meta-Networks with Overlapping Autonomy
Examine it in its external environment and you
will discover it is intimately networked into ever larger systems of
systems and networks of networks. This interlinked cross--boundary
meta-network structure of relationships is intrinsic to how the
self-organizing autonomy of networks emerges at larger and larger
scales. A baseline level of a human body/mind system includes not
only billions of cell systems but also a vast variety of
micro-organisms that live in and on the body which are integral to its
overall functioning. Human digestion is not possible without billions
of diversified bacteria living in the gut. Depending upon what
food a person ingests these organisms will, in performing their own
internal metabolic operations, generate byproducts that have different
chemical properties. These in turn effect the operations of the body's
organs. The bacteria constitute discrete systems and networks, yet they
are intimately interdependent with that of the human body. Each
manifests discrete aspects of network autonomy. These overlap and yet are some
times in conflict, as with bacterial networks that are part of our body's systems but can also disrupt body
functions.
Individual birds in a flock or fish in a shoal have considerable
autonomy. Each seeks to avoid predators. But by synchronizing their movements
they patchwork together to form a single meta-network that frustrates a predators efforts to single one out for attack.
Simple rules that create complex network behavior
Fish following cues from a few neighbors generate emergent meta-network autonomy:
Termed "murmmeration," this effect derives in a bottom-up manner through the
correlation of separate networks, the individual autonomies of the fish,
in which each copies the movements of its nearest neighbors. Yet when a
shoal is being pursued
by predators. individuals will sometimes break with this collective
effort and select to act independently from the group. All this occurs
as each autonomous network processes information about others in its
environment then acts in ways it selects as beneficial to itself.
The collective effect is a dynamical example of networks operating in
criticality near the edge of chaos. The meta-network of the shoal is
constantly in flux, re-organizing itself continually on the verge of
fragmenting and reforming. Such is the teetering instability at the basis of complex network self-organizing criticality.
Meta-system network autonomy manifests in the
collective system interdependency of ant colonies as well as human
societies. These are called agent-bases systems and constitute what are
termed super organisms because the manifests networked intelligence
that derives from group interactivity. Yet this larger scale emergent
network is not a thing that can be specified or seen. It is a being
with no particular substance. It is only made evident by the emergent
properties of collective behaviors that, in their particularity, are
not it. Such networks are ethereal fields of information exchange and
processing without any central nervous system or control function.
Their self-organization effectively animates their systems in ways that
produce characteristic but unpredictably adaptive behavior.
Similarly, each
individual plant and animal in
an ecosystem, such as a lake, is a system of systems that itself
interacts interdependently with others. Microbes live interdependently
inside of fish, fish feed on insects, birds on
fish, bird feces fertilize plants that foster the insects, and so on,
round and round--but also "all at once." Such
activity can appear to be competitive and hierarchical. But actually
there are so many interdependencies these cannot be fully mapped
out in logical sequences. It is a continual "dance" involving
innumerable simultaneous mutually modifying interactions--one that is
logically unpredictable because it could not exist otherwise.
Below is a general but misleadingly simple
scheme of an ecosystem food web network. Each of the creatures pictured
is itself a complex system whose sub-systems have interdependent
relationships with those of the other creatures and with their shared
external environment that are so complex and variable as to be
un-representable.
Many simultaneous interactions create meta-network self-organization
Various species influence each other in reciprocal flows of feedback
that collectively manifest a self-regulating system:
Such sets of
relationships, due to their intricate interdependencies and the
selective actions of their network autonomy, are in constant flux over
time. So the
best description of this vast reciprocal interplay is to call it
something like a dynamic patchwork that aggregates into a fluctuating
meta-system,
since it does not have a clearly definable, much less consistent
network structure. These relationships have also been referred to
as nested system to indicate that their boundaries effectively overlap and penetrate each other.
So despite their interlinked reciprocity,
there
is autonomy at work in all sub-system components of a meta-system or
nested network of systems--out of which a collective network autonomy
emerges. Each species is
composed of many individuals but these together constitute a sub-system
of their ecosystem. Network autonomy manifests at both the individual
and collective level of the species. When one species network behavior
changes, others autonomously respond. This is not to say that these
networks consciously calculate changes in their operations as do
humans. And obviously, some changes in a system are imposed from the
environment: declines in food recourses can directly cause declines in
the population of even survival of a species.
But there is more to the
overall sustainable self-organization of an ecosystem than these
seemingly mechanistic effects.
There is ample evidence that specific systems, both individual animals
and their collective networks somehow track and respond to
environmental changes in ways that promote their continued
sustainability--a condition indicated by the term super organism. Thus in some regard, networks experience them
selves within their environments and act volitionally in response.
However, just where the autonomy of one system network leaves off and
another begins seems ultimately impossible to differentiate, due to
their radical interplay that creates so many overlapping domains of
network autonomy, all of which feedback into each other.
In so doing, larger and large scales of
aggregate meta-networks emerge from sub-system autonomy, resulting the
extended horizontal range of reciprocal relationships that constitute
the meta-systems of connected lakes, forests, and deserts, or of
persons, neighborhoods, cities, and nations. The topology of such
extended meta-networks have the structural characteristics of hub
configured de-centered models and of the even more interdependently
connected distributed ones. Despite our best efforts to model and
calculate these relationships, it is fair to say they are beyond
literal description.
The Logic of Patchwork Hierarchy, Higher Level of Operations, and the Limits of Command and Control
Patchwork interdependencies of networks can
also be viewed as generating more hierarchical levels of influence.
Individual body cell systems are networked together in a variety of
ways, depending on cell system specialization, from skin to gut to
brain cells, each meta-system having some autonomy within the body but
also collectively generating higher level whole-body operational
network that, having emerged from this bottom-up networking, can exert
some command and control influence in self-organizing the larger system
in a more top-down manner. But the higher level operation does
not so much control as integrate the autonomous operations of the
sub-systems from which it emerges. So it is important to realize that
the higher level operations are always part of a reciprocal flow of
feedback among those sub-systems, which function as hubs in the overall
body network, whose structure is best represented by the de-centered
type of network structure topology.
This view of the body/mind system seems to
contradict a common assumption that the body regulated by a top-down,
command and control operation of the central nervous system, directed
by the brain. But despite the seemingly hierarchical structure of that
neurological system, and its evident aspects of control over many
aspects of the body's component systems, it also needs to be regarded
as a vast feedback network for in-puts and out-puts of those
subsystems, which are the bottom-up basis of its higher level
operations. Ultimately, such structure does not lend itself to directly
controlling top-down control by higher level operations.
Larger meta-networks like ecologies and economies
are even less hierarchically networked than an individual animal body,
lacking a central nervous system. Thus these yet less susceptible to
top-down manipulation and control. In fact, when humans attempt to
impose such control on these types of systems the result is that their
own self-regulation, their own network autonomy, can be disrupted and
cause them to collapse into more chaotic dynamics.
Given these references for network dynamics,
it becomes important to be wary about assuming systems are as we humans
tend to think they are--or would prefer them to be. Even simple seeming
personal relationships constitute an interplay of systems from which a
meta-networks of autonomous operations emgege: two makes for the crowd
of three.
Two networks make three that are one
The interdependent interactions of a seemingly simple two-person relationship
generates an additional, autonomously intentional meta-network:
Similarly, larger groups, like
families, corporations, and governments, might appear to be strictly
hierarchical structures with absolute command and control networks. But
in actual operation, these tend to be complex systems whose network
operations arise from more distributed connectivity and interdependency.
Appearances are deceiving
Despite hierarchical design, real world systems tend to self-organize
emergently, through distributed connectivity:
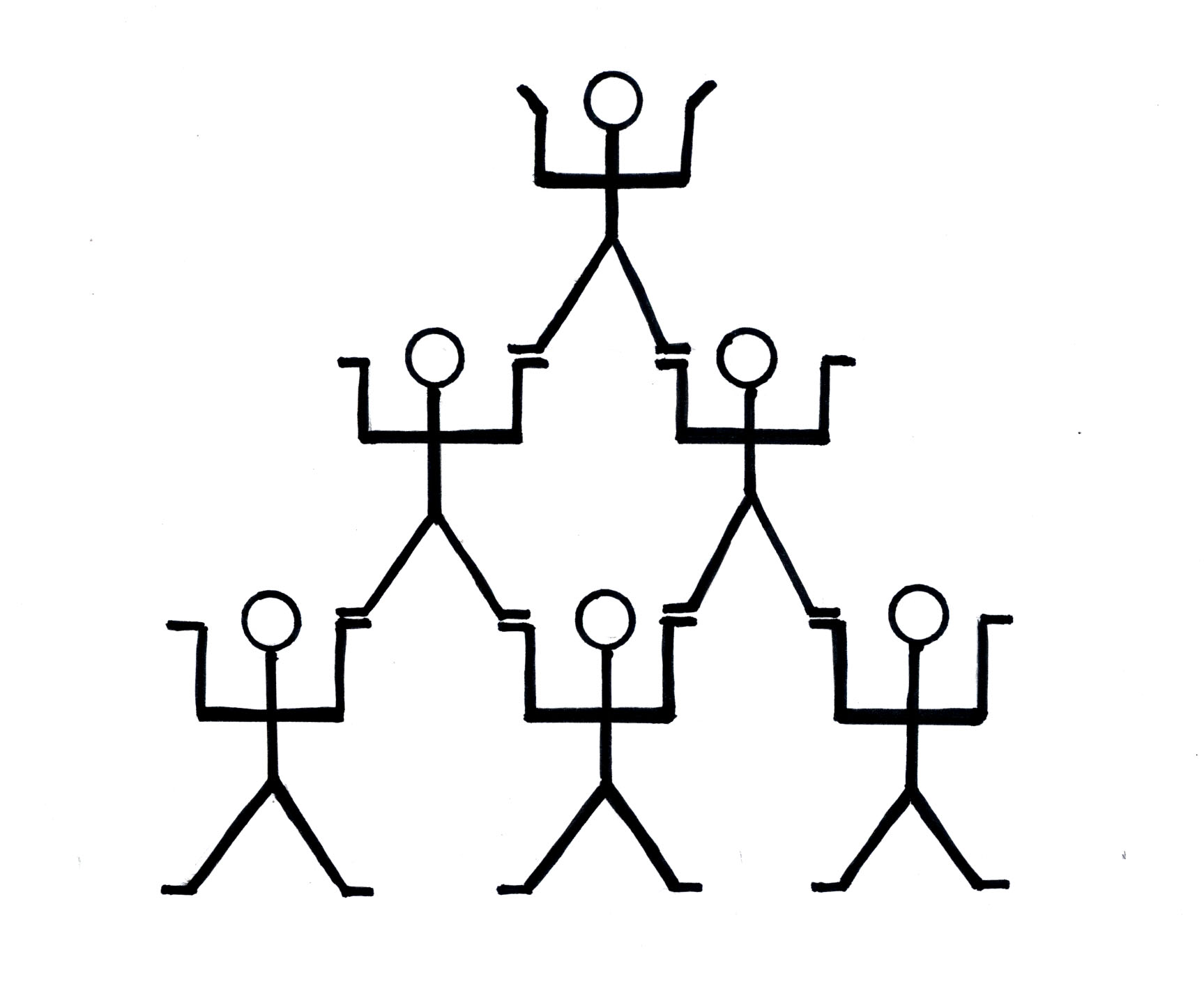
Our
human proclivity for command and control over our selves and our
environments leads us to see the world in sequences of predictable,
thus controllable cause and effect. Our pragmatic interests seek the
world in hierarchies of controllable cause and effect. But network
science now cautions us
about the dangers of such presumptions. Nature is more hetero-archical
than hierarchical.
Hierarchical projection on hetero-archic reality
The competitive, control obsessed perspective of the human "ego"
misperceives the patch-work dynamics by which Nature actually acts:
Here it is worthwhile to
remember that what we can directly perceive of a complex system is not
its operational network. What is accessible to our perception, even
under the microscope, is not a complex system's dynamical
interdependency. The familiar singularity of entities that are in fact
manifestations of complex networks, whether a person, a forest, or a
city, is an illusion. What makes these the way they are is hidden from
our ordinary awareness, partly because we do not practice "seeing" them
as networks and partly because their dynamics are effectively ephemeral.
What is evident is not all there is
Nature's complex adaptive system networks create the majority of order in
and around us--but remain effectively invisible to direct observation and calculation:
The Logic of Ordering Out of No-Where: The Betwixt and Between of Network Self-Organization
The contrast between the predictably
structured ordering of mechanically linear dynamics and the seemingly
anti-structural order creation of complexity's nonlinear ones gives the
impression that the latter "comes out of nowhere." The absence or
progressively proportional causal sequences leaves us with no causal
path to follow. There is no specific origin or trajectory to the events
that result in emergent properties and self-organizing networks.
De-centered and distributed network models assist in imaging a field of
interactivity between system parts, and systems, as a kind of spatial
zone of emergent causation. But this is a betwixt and between place
without a chronological sequence. Many events are occurring all at once
but we cannot account for all of them in physical terms. Much of the
feedback involved is constituted by the processing of abstract
information that does not have concrete physical properties.
Thus the ordering that results does not happen
either in specificable places or times, and so has not center or
structural hierarchy. It "takes place" in what can be termed a metaxy,
a placeless place of in-betweenness of interactions among systems parts
and the system's external environment. It is not even defined by
evident system boundaries because inputs and outputs are continually
flowing between the system and its environment of other systems. This
external field of in-betweenness effectively extends a systems network
beyond itself, which leads to the nesting of multiple systems into the
emergence of larger scale meta-systems.
Where then can we locate the nexus of control
over the operations of a complex network? It has no single center and
seems to be everywhere and no where. If appears to have immaterial
aspects, like a thought or idea, yet clearly has causal effects on the
physical world by organizing and re-organizing its forms "at
will." Network operations change their systems and that changes
their environments and that prompts network changes and round and round
it goes. However, returning to network modeling, that is just what we
see in the connectivity of de-centered and distributive network
structure: continual flows of interdependent feedback with no
beginning, middle, or end. It is logical that the network
self-organization this ultimately undefinable metaxy that is "neither
here nor
there."
Despite our inability to de-construct the
resulting dynamics as causal sequences, if we aim to have a sense of
how the world actually works, we must derive some sense of how this
activity "does what it does" without having a center or even being an
identifiable "thing." Like it or not, this unstructured phenomena
generates more and new structure, indeed most of the biosphere's
structure. That is profoundly important.
The Logic of Networks Beyond Control: The Logical Independence of Network Autonomy
Complex adaptive systems are
distinguished not only by their unpredictable emergent creativity but
the impossibility of deliberately controlling their operations. It is
one thing to influence or to some degree manipulate those operations
but quite another to take control of these. Again returning to the basic modeling of network
structures and dynamics, it becomes obvious why this is true. In so far
as emergent causation and self-organization have no distinct structure
or sequencing, there is can be no way to directly manipulate and
control their operations. In
network science, it is logical that there can be no way to directly
control network autonomy as we do mechanical systems. This is because
there is no predictive structure, no mechanisms, to control. Complex
networks are beyond control. But attempting to do so certainly has
effects on the capacity of complex networks to maintain their self-organizing, self-sustaining operations.
Our technological powers
enable us to exert massive influences on complex networks, as we do the
human body/mind system through medications and surgery, or ecosystems
with deforestation, damming rivers, agriculture, mining, and urban
development. Such manipulations gain us short term advantages, but as
we are now learning worldwide, the effects on natural systems are
creating overwhelming problems for them, and thus ourselves. We seek
control over natural systems, as we do our human generated ones. But
even our human systems have proved to be far more complex than we
understand.
Network modeling involves highly specialized
mathematical techniques that reveal the nonlinear dynamics involved.
This mathematical analysis assists in confirming the unpredictable
effects of network autonomy and documents the impacts of human
manipulations on natural systems. One concept involved is that of
tipping point. Complex system self-regulation is paradoxically
both impressively robust in the face of environmental changes
over time yet also surprisingly vulnerable to the slow accumulation of
interference in the reciprocal flow of feedback between system parts
and nested systems. Human activities change environments at
speeds and in degrees that readily disrupt the capacity of natural
systems to adapt to the effects.
Effects like the introduction of massive
levels of phospherous fertilized to ecosystems from farming or CO2
gases from fossil fuel burning into the climate systems gradually build
up interference in the feedback of inputs and outputs in those systems.
Eventually these effects finally overwhelm network autonomy, creating
what is termed a tipping point. The term indicates that a system is
pushed beyond its dynamical domain of self-organizing criticality near
the edge of chaos and "tips over" into chaotic dynamics. The result is
termed cascading collapse. There is no "turning back" once a tipping
point is reached. Systems subsequently become highly irregular and
often disintegrate all together. The failure of one system can lead to
the collapse of an entire meta-system network in a domino effect.
Eventually new forms of systems will
regenerate through the emergence of new meta-system networks, but only
if there a few survive to form the basis of the regeneration of
complexity. Large scale examples of this are found in mass extinctions,
such as that of the dinosaurs, and in human social revolutions
resulting in the collapse of political and economic institutions.
Currently in the 21st century, human activities have been documented as
producing these dramatic tipping points across global ecologies and the
entire planetary climate system. Believe it or not, from the
perspective of network science, we have disabled the self-organizing
network autonomy in natural systems at a speed and to a degree that has
almost no precedent in the geological record. The future will be, at
best, wildly unpredictable and erratic.
The Logic of Network Autonomy Being the Minding of Matter
The thought that
network self-organization emerges "out of nowhere" yet orders the
biosphere is unsettling to our mechanistic perspective. If it does so a
manner similar to how immaterial thoughts or ideas can have physical
effects by prompting human actions, it is tempting to think of it as a
form of "minding" in Nature. When it comes to the mental system aspect
of a
person, this can seem like an overtly top-down command and control
operation, as in "I do what I tell myself to do." However, the physical
brain system and network from which
mental activities emerge is itself a network of interdependent,
mutually
modifying networks. The brain areas associated with logical
reasoning and emotional feeling are significantly independent yet also
have been shown to be interdependent in facilitating each other. The
nuerolgical network structure of the human brain is so complex it is
regarded
by some as the most complex entity in the known
universe. But what then of the mind that emerges from it?
Psychological analysis reveals that mental
activity has a similarly diversified composition, with many mutually
modifying, often conflicting relationships, the conflicted co-operation of which collectively
constitute its ever variable totality. Though standard definitions of
causality based on physics lead us to assume that our mental systems
must be directly derived from the properties of matter and energy,
network science puts this assumption logically in doubt. Furthermore,
despite
many serious efforts to prove that mental activity is identical with
physiological brain activity, compelling evidence for this idea has not
resulted. Similarly, attempts to duplicate human mental capacities
using computational machinery, as artificial intelligence, have not
been even remotely successful. But if mind-ing is not "in the brain,"
where and what is it? And is this ability to interpret abstract
information and respond to it by creating new forms of organization, of
invention, and willfully acting to create it, exclusive to humans, or
animals?
From the perspective of complex adaptive
systems science, human mental operations appear as yet one more, if
perhaps the most extreme, case of higher level network autonomy arising
emergently as self-organizing criticality from a the radical
interdependency of a system operating near the edge of chaos. The brain
constitutes the physical meta-system of a collective collective of
sub-systems, each with significant network autonomy, whose
simultaneously interactive, mutually modifying
interdependencies emergently generate a higher level network
operation--which we call mind or
consciousness. Just as with other natural systems, this higher
level network is not the same as the physical system from which it
emerges, despite the fact that it can be shown to influence the
activities of that system in turn. Brain and mind are two differentiable yet interdependent meta-systems.
The minding of matter
"Lower level" interdependencies in the material brain emergently generate the
"higher level" psychic system, whose network autonomy in turn influences
that of the brain--forming a meta-system:
Thus
there is indeed
scientific evidence to regard physical matter as the basis for the
emergence of mind as a more than material, and indeed, other than
mechanical phenomena. This evidence is derives not only from our
inability to reduce human thought to physiological brain activity. It
is found across the spectrum of complex adaptive system networks in
Nature. The evident criteria for this pervasive, if ultimately
mysterious,
emergence of an at least partly immaterial network agency is the
presence of sufficient dynamical complexity--a complex network
manifesting self-organizing criticality. In this regard, the
evidently extreme agency and intelligence of human mental networks
associates with a similarly extreme level of complexity in the physical
system from which it emerges, meaning the brain. Mind in this view
equates with the general concept of emergent network autonomy across
the vast diversity of complex adaptive systems.
There is reason then to
associate the characteristics of network autonomy in a single cell
organism, a forest ecology, and a human, as a single category of
phenomena. Different as these seem, network analysis shows them to
constitute a spectrum of effects or properties that can be scaled up
through increased system and network complexity--reaching their evident
extreme in humans. The differences between "us and them" appear to be
more a matter of degree or scale of complexity.
This notion of scalable capacity for
autonomous behavior and intelligence suggests that there is some form
of consciousness active in systems that are not composed with central
nervous systems and brains. Even in regard to humans, there is much
argument about just what consciousness is and even whether volitional
free will exists. Evidence from brain research indicates that most of
cognitive mental activity in humans is "un-conscious," in the sense
that one is not overtly self-aware of most of what one is thinking and
even feeling at any given time. There are further indications that what
we regard as self-conscious decisions might actually be determined in a
pre-conscious manner, meaning through cognitive operations that occur
prior to one overtly knowing one has made a decision to act. Such
evidence is cited to argue that we do not actually have volitional free
will.
From the perspective of network science, network autonomy appears as an
empirical fact detectable in even non-living systems, judging from its
quantifiable effects, despite the limitations on describing and thus
explaining its emergent dynamics. This suggests it need not be overtly
self-conscious in the manner we experience it. Thus, even if human
mental networks operate in a largely un-self-conscious manner, meaning
below a threshold of overt awareness that these are happening, that
fact does not disprove that such networks manifest autonomous
self-organization and thus volitional behavior. Psychological analysis
was created with Freud's theory of a conflicted domain of mind that has
been repressed "below the level" of overt self-awareness, yet exerts
pervasive influence over every person's behavior. Such observations of
a sub-conscious autonomy are commensurate with network science:
volitional network autonomy does not require a brain, much less
demonstrable self-reflective awareness that an entity is deliberately
making decisions.
Thus it seems logical that
the overtly self-reflective awareness of human mental networks is just
one more emergent property of complex network autonomy, rather than
being a definition of it. During sleep, our overt self-awareness is not
evident, yet our mental networks continue to manifest autonomous
creativity in the form of dreaming. So what ever consciousness, human
mental networks have the archetypal traits of network autonomy as it
apperas throughout natural systems.
Thus there
is reason to conclude that network autonomy constitutes "the minding of
matter" in a scalable manner. As a basic emergent property of complex
systems its effects on system behavior appear across a stunning variety
of systems, animating each in characteristic ways. But it can arise
with additional emergent properties deriving from additional complexity
in particular types system networks. From it is "at work" from the
self-organization of ecosystems to the super organisms of ant colonies,
and single cell plants to the vastly amplified complexity of human
brain/mind meta-system networks.
The more complexity that a system can
manifest, the more potential there appears to be for elaboration of its
emergent properties of information processing. The scaled up complexity
of animal systems associates with what we recognize as volitional
intelligence. The greater a type of system's complexity, the more
likely it seems to be to produce individualized versions of its
characteristic autonomy of these. Individual ant behavior is difficult
to identify but different humans tend to manifest differentiated
qualities of human mental network operations. Whatever consciousness
is, evidence for network autonomy and its variable qualities of
volitional action that animate complex systems throughout Nature is
compelling.
The Logic of Archetypal Dynamics in Network Operations
The most basic archetypal traits of network dynamics
Archetypal
Mechanism
Archetypal Complexity
A basic electrical circuit network:
A complex social network:
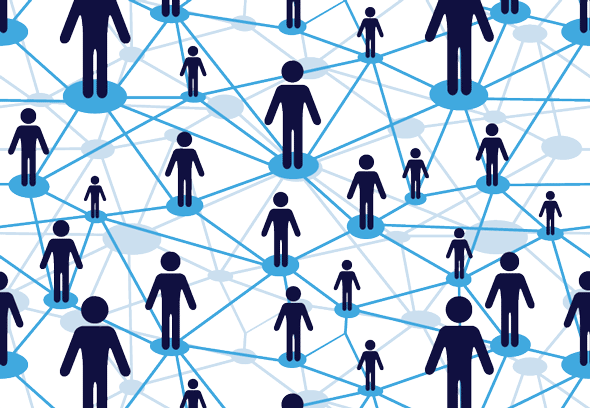
Diversity is an
essential
quality of complex systems. Different types of parts with different
properties and various configurations of connectivity are required to
generate their complex dynamics, and thus their particularized network
autonomy. These
differences necessarily involve elements of conflict between parts and
their actions. Thus contrast and potential conflict appear essential to
the emergence of self-organizing operations of network
autonomy. The latter is not produced by systems of composed of similar
parts that act on each other in hierarchically sequenced, dependently predictably, proportionally
consistent ways, as in machinery.
This diversified basis for network
emergence has some pre-existent references for how it takes form. There
are recognizable types of systems we can distinguish by both their
structure and activity--or behavior. There are systems with primarily simple linear
dynamics whose parts act upon each other in predictable ways, such a
mechanical ones. Then there are systems with complex nonlinear
dynamical properties whose parts act on each other in unpredictable yet
order-creating ways. We can think of these a two
fundamental types or "arches," meaning an original form. Thus we can
think of the basic types of systems such as animals and ecosystems,
then more particular ones such as birds and deserts.
Complex systems are especially prone to
exhibiting variations of both form and dynamical patterns of activity,
or network behavior. No two complex systems are identical. There are
many types of bird species with particular behaviors, many types of
desert ecologies. This variability extends down to each individualized
system. Pelicans are recognizably similar manifestations of their
species type, but each individual manifests some aspects of uniqueness.
High elevation deserts tend to share some traits of form and function,
but each is somewhat different. This variability on a theme appears
logically consistent with their basis of diversified parts producing
partly conflicted or disorderly interactions that are also
interdependently interactive--which are the source of their emergent
self-organizing network operations. It makes sense that these aspects
of system configuration and activity result in their dynamic
variability over time, as they self-organize and re-self-organize in
response to changes within and outside of their boundaries. This
on-going variable behavior means they are not identical to
themselves from moment to moment and can even undergo dramatic
reconfiguration yet still be identifiable as a type of system or network
behavior.
Thus we can think of systems as having traits
that characterize both their general type and their individualized
expression of that type, both their forms and behaviors, even their
unpredictable yet characteristic behaviors. We cannot predict what a
particular bird species or single bird will do next, but we can get a
general sense of how these types of systems tend to take form and
behave through their network self-organization. These characterizing
traits can be thought of as archetypal, in that there constitute ranges
of characteristic behaviors associated with a type, rather than
definitive categories. There is a typology of network formation and
behavior, but it is not fully predictive. Complex systems and their
emergent networks have archetypal character, both generally and also
specific to each type of system and each individualized manifestation of
that system type. An
animal species is not a fixed thing but a collection of characteristic
behaviors which, though recognizable over time, are also constantly
variable and adaptive through their archetypal network autonomy.
Whales are a type of animal
system that manifests as various sub-species with distinctive traits.
Individual whales of a given sub-species can be distinguished by
physical and behavioral traits. This individualization of basic types
of systems is evidence of the infinite creative capacity of complexity,
acting within the constraints of the Laws of Physics, but not evidently
bound by these in terms of how it creates such diversity of original
forms of organization of systems. Different types of trees branch in distinctive patterns. Maple
tress are identifiable by their archtypal patterning of branching. But
no two maples branch in exactly the same pattern. There is an impetus
toward maple branching, but every individual maple tree network
autonomously directs its particular version of that impetus.
A given system can manifest
with a variety of archetypal traits, which, taken together,
characterize the individuality of its particular system formation and
operational network autonomy--or behavior. A city might be structured
and function in an more archetypally hierarchical manner, with a
distinct center and well regulated traffic, while another might have no
identifiable center and chaotically unregulated traffic. Both are
archetypally cities but express that type with different archetypal
traits of configuration and operation.
So the dynamical processes that create systems
and their emergent networks can be thought of as archetypal tendencies
of formation and self-organization--types of ways that things happen in
a world of complex systems. These are both physically formal and
dynamically behavioral.
A humpback whale is an expression of the archetypal tendency to form
such a system. The operqtional network autonomy of such a system has
archetypal character. But a given individual manifests that tendency in
somewhat unique ways, as a particularized expression of the range of
potential in the general archetypal tendencies of the humpback system
formation. Thus the archetypal character is expressed both by the
general tendency and its particular manifestations. One humpback
might be more aggressive than another. Such behavioral traits lead us
to the notion of the psychology of network autonomy.
Given this view of archetypal traits of formation and
dynamical tendencies, network autonomy can be regarded
as having particularized qualities of
self-organizing impetus that are both generalized and
particularized. This archetypal character of any given network
constitutes the personality or its autonomous behavior.
The Logic of Networked Reality: Complex Network Autonomy Animates Self and World
These basic aspects of network structure and
dynamics provide the scientific basis for a radical shift in our
understanding of reality. We have been taught a basic contrast between
mechanically orderly and disorderly dynamics. But now we see that there
is not simply a spectrum from ordered to disordered, from predictably
deterministic to unpredictably random. There are also dynamical
conditions that deterministically create order out of significant disorder--and do so unpredictably. Similarly,
standard physics-based scientific explanation describes the universe as
progressively less ordered or entropic, according to the Second Law of
Thermodynamics. Overall the universe is viewed as inevitably, inexorably, becoming more disorganized and random.
However, complexity has been shown to generate
new and
likely infinitely variable forms of organization, despite the assumed
entropic trajectory of the universe as a whole. The evidence suggests
that Nature has some innate impulse toward creating greater and more
diverse forms of emergent complexity. This fact further
confounds our efforts to explain how such additional ordering is
created. From the explanatory perspective of physics, it "should not"
be happening. Thus arises the notion that there are actually two
different
modalities of order creation, that of the Laws of Physics and that of
complexity's emergence and self-organizing systems. Though causally different,
these two types of order creation must also be co-arising phenomena of Nature. Needless to say, this is
not a popular concept in the realm of contemporary science.
Nonetheless, we are confronted by compelling
evidence that things happen in ways we can't fully understand. Despite
the knowledge
provided by network science, we are left without any scientific means
of fully analyzing the causal creativity of complexity. How are we to
think about it in ways that give us further insight into its crucial
role in our existence? How are we to "see" the world "the way it
actually happens"--as neto-logical reality?
It is evident that this creation of order out of
disorder turns out to be the source of most of the form and
organization that makes up our selves and the biosphere around us.
Through a vast unplanned, interdependent interplay of simultaneous,
partly chaotic flows of outputs and inputs, acting as feedback within
and between systems, networks form that imporvisationally self-organize
to generate otherwise impossible variations of complexity and novelty.
Species evolution could not occur without this emergent creativity, if
only because the behavior its autonomous networks create influences the
environments within which natural selections occurs.
In the
logic of networks, it takes disorder to generate the complexity that
can emergently create networks that act autonomously and purposefully
to produce the manifest complexity of forms and behaviors that
constitute humans and the world we inhabit. At varying scales of larger
and larger meta-networks, network autonomy processes information about
its systems and their environments in ways that equate to a form of
thinking. Subsequently they act as if alive, whether or not they are
composed as biological entities.
Furthermore, nearly every thing one encounters has
in some way been influenced by the self-organizing autonomous networks
of complex adaptive systems over eons of time. We exist in an oxygen
rich atmosphere because of the complex adaptive systems of plants,
whose particular archetypal mode of operation utilizes the in-put of
carbon dioxide and generates the the out-put of oxygen. That out-put
enabled the evolution of the archetypal typology of animals, whose
systems use it as input to their operations. And that oxygen has myriad
chemical effects even on the geology and meteorology of the physical
planet.
A house is a specifiable physical structure
that an be explained in the terms of deterministic physics. But this
analysis tells us nothing about how it came to have its form. That is a
product of autonomous network intentionality. A plane flies according
to the laws of physics but it only exists, thus flies, because network
autonomy emergently organized its mechanical system parts into its form
and the function of flight. Complexity may be an emergent property of
physics, but its autonomous networks have the capacity to organize
physical matter and energy in ways that could not exist otherwise.
Those emergent properties become part of the physical world that in
turn is the material from which complexity generates yet further
emergent properties.
Network autonomy gives form and function to
the physical systems from whose interdpendent dynamics it emerges. It
effectively makes these animate entities with volition and archetypally
characteristic behavior.
The Logic of Network Character: The Archetypal Traits of En-spiriting Information in Network Self-Animation
The archetypal diversity of autonomous system
networks and their reciprocal interdependency is the creative basis of
the biosphere. The archetypal tendencies of forming and behavior we can
identify across this vast networking of networks are the expression of
each system's autonomous self-organization that effectively animates
that system by directing, and re-directing the behavior of its parts as
it interprets information about itself and its environment in each
moment of time. Furthermore, these networks accomplish this by
incorporating information about their history to act willfully, with
the teleologically purpose of preserving or adapting their system for
sustained survival. Thus we can regard our selves and other complex
adaptive systems as intentionally self-animating entities whose
interplay animates the biosphere as a collective meta-network.
that are identifiable by our archetypal characteristics.
Though we cannot analytically determine exactly how these
networks function, we can differentiate aspects of their dynamical
behaviors as tendencies to manifest types of form and activity--their
archetypal character. These archetypal tendencies have a history in the
emergence of past system networks that present one can somehow
improvisationally re-generate from the partial instability of each
present moment. Systems somehow use this information in
establishing their particular manifestation of their type of archetypal
forming and behavior. But by doing so in an individualized way,
combining element in various and novels ways, each adds some ela
boration to the tendency, and that variation on a theme can become
relevant information to the operations of other systems.
In biological systems there is an evident
storage for historical system forms and operations in genetic encoding,
though this information must be accessed and interpreted by emergent
network operations relative to its systems current conditions. And much of network operations in animal systems derives from
learning by interpretation of interaction with other systems and
environments. But
many complex adaptive systems, such as ecologies and cities, are not
constituted with either an encoded genetic memory nor a central nervous
system and brain. Nonetheless, these generate form and behavior having
archetypal character related to others of their general type which
cannot be predicted or explained in the terms of mechanistic physics.
Again, the physical structures of cities and forests can be fully
analyzed and explained, but now how these came to be organized in the
way they are. Their actual network self-organization remains a black
box of dynamical activity into which we have not scientific perception.
In manifesting typical yet variable patterns
of archetypal character, both animal systems and non-living ones give
the impression that their pattern creation of from and behavior is
being influenced by past tendencies. It is as if there are originating
types of formation and behavior that influence how things take shape.
These types of forming tendencies are neither fully deterministic nor
explanatory. They are
only descriptive. In addition, such tendencies evolve over time through
emergence of variable versions. They taking on new traits
while often remaining identifiable, whether as succeeding generations
of a family line of humans, a species of frogs, or the successive
reconstructions of a city.
It is at this point that attempts to
understand such phenomena prompt the notion that the network
self-organization that effectively animates the systems of the
biosphere is in some manner in-formed or guided by what, for lack of a
more scientific term, seem to be the in-forming impetus of archetypal
spirits. It is as if system formation and network operations are
en-spirited by a diversified range of archetypal tendencies, to which each individual network gives some unique expression.
In that regard, every system and network's
self-organizing animation is
an expression of en-spiriting archetypal information. Or rather, a
whole range of diverse, even conficting archetypal traits that,
manifesting interactively within and as a particular network, give it
its diversified individuality as an animating
spirit of archetypal information. Which is to say, a complex of
archetypal tendencies configures
the characteristic personality of whatever goes on inside the black box
of an emergent
network. As vague as it seems, it at least gives us a way to think
about the ephemeral operation of network autonomy. It also assists in
comprehending the correlation of
network science with mythical symbolism as the metaphorical version of
complex network modeling.
A Netological Philosophy: Thinking Like Nature Acts through Two Types of Network Dynamics
It is possible to distill a basic
philosophical perspective on reality, or the way Nature acts, from the
bi-dynamical knowledge of order creation in systems and their networks.
There are two different ways in which forms and functions come into
being, two dynamical aspects to the ways that things happen. These
associate with two types of network structure and their related
dynamical activity. One emphasizes the predictably deterministic,
structurally consistent, progressively proportional causal processes
associated with the Laws of Physics. The other emphasizes the
un-predictably deterministic, structurally inconsistent, progressively
disproportional events associated with complexity's emergent
creativity. We might call these structurally dependent versus
interdependent network dynamics. This distinction is made in regard to
the contrast between structure or order that results dependently from
preceding or past conditions and that which does not, but rather from
the interdependency of interacting of factors in the moment .
This view generates a bi-dynamical conception
of order creation: the predictably mechanistic and the unpredictably
emergent. Both are intimately involved in the formation of nearly all
aspects of the biosphere. But that reality cannot be remotely
comprehended without a sense of the differences between the two.
Learning to think in terms of network structures and their related
dynamics gives us a starting point to increase awareness of how things
actually happen. This is necessarily an abstract effort, since not even
the networks associated with mechanistic causation are overtly obvious
to perception. One must conceptually take systems apart to identify the
networks of even many simple mechanical systems. When it comes to
complex systems, analytically taking these apart does not fully reveal
the actual dynamics of there networks. Frustratingly, even for the most
advanced scientific methods of analysis, complex networks remain at
least partially "invisible."
However, making the effort to distinguish these types of
networks informs us that we are indeed confronted with the phenomena of
syntergistic emergence, rather than only mechanistic determinism. As a
basic philosophical tenant, this notion of reality arising from two
types of network dynamics, directs us to become more constantly aware
of the roles they play in our selves and the world. We are and inhabit
a realm of invisible complexity. Thus it is useful to always be asking
one's self just what type of systems and networks one is trying to
understand or relate with. We would be wise to continually ask
ourselves, "What type of networks am I looking at?" The simple
contrasts in network structures are a kind of iconic reminder of these
differences.
Mechanistic Netology
Traits of network structure associated more with proportionally consistent
structuring of predictably mechanistic events:
Simple sequential
icons for progressively consistent events in which each proceeds
from
the previous, as in 1,2,3, or all converge in an identifiable causal
process:
Complex Netology
Types of network structure associated with the proportionally inconsistent
structuring of unpredictably synergistic emergence:
The greatest challenge comes in
attempting to iconically represent the dynamics of interdependency and
emergence. Somehow we must prompt ourselves to be aware of the
in-betweenness of each moment's interactions among feedback flows, of
an instantaneous back-and-forth that is an every-which-way and
round-and-round of mutually modifying factors. Inadequate as
progressive modeling is to represent such a notion, it gives us a start
at conceiving the invisibility of complex network dynamics.
The iconography of interdependency
Graphics for suggesting the instantaneous interdependency of interactions:
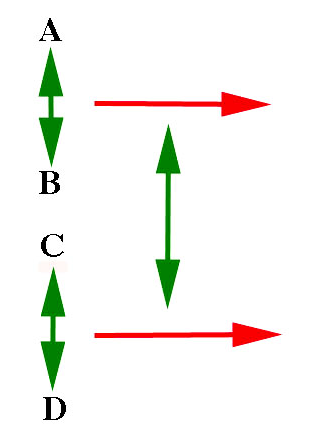
We can also attempt to represent a time sequence of
moments of instantaneously interdependent interactions having
disproportional consequences, as if the sequence were 3,4,2:
But to attain a more apt impression of such interdependent dynamics in
each instant, and ongoing over time, we need to abandon our focus on
sequences. This invisible interplay between factors is given a visualization
in the the turbulent flows of interacting colored fluids between multiple vortexes, shown below.
The latter represent system parts, or interacting systems, and the
mixing between these the interdependency of mutually modifying network
operations. The image on the left below gives an impression of a particular instant of interactive
dynamics. Figuratively, it is like a snap-shot of meta-system interdependencies, with
feedback influences from many interacting systems flowing out and mixing to
generate the network operations of a larger meta-network. The image on the right gives a sense of the difference between predictably sequential actions and the turbulence of the ongoing evolution arising from mutually modifying interacting ones over time.
The logic of disorderly ordering
Turbulent mixing and linear or laminar flow versus turbulent flow:
The Logical Mystery of Emergent Network Order Creation
However, despite the science behind this logical
notion of differentiating network dynamics as dependent versus
interdependent, and the basic philosophical
perspective it suggests, we are left with a kind of void. There is no
conclusive empirical evidence to confirm logical speculation on just what
actually happens in the
in-between realm of interdependent dynamics and emergent order
creation. This limitation on how precise our understanding can be is
crucial to any realistic perspective on how things actually happen, and
thus how Nature acts to generate the biosphere.
Indeed, reasonable reflection upon what
scientific method can reveal about ordered forms emerging from chaotic
dynamics and self-organizing networks from complexity's state of
criticality, readily prompts a sense of the miraculous. Firstly is the
implication the the predictably deterministic order creation of
material physics is the basis for the unpredictably deterministic order
creation of complex dynamics. Secondly, there is the conundrum of this
unpredictably, thus inexplicably emergent ordering to self-sustain its
forms in a self-similar manner over time through self-regulation,
despite perturbations, and even act willfully to adapt its forms and
operations for the purpose of maintaining the existence of the system
from which it emergently manifests.
From the perspective of material physics,
these are confounding facts. Nonetheless, most of the forms and
functions we are and surround us have been shown to arise emergently.
This appears illogical until once closely contemplates the differences
in linear and nonlinear dynamics, in orderly order creation versus the
disorderly conditions of self-organizing criticality. If the latter
dynamics are creating order, then it would logically be of an
inconsistent and unpredictable character, whose identifiable patterns
could not be exact reiterations but only self-similar variations over
time.
That being the case, then the very
existence of the order we are and inhabit, deriving from such dynamics,
is logically mysterious. It can come into being, and be sustained, only
through dynamical conditions that are inscrutable our methods of
precise quantification and calculation. To exist, it must be mysterious
to a mechanistically causal explanation of events and transformations.
The point here is, that though such a conclusion is deeply
dissatisfying to our physics-based, pragmatically control-oriented
worldview, it does have a reasonable basis. And there is yet more
science to elaborate this conundrum.
The Logic of Networks as "Dynamical Attractor Landscapes"
Scientific analysis of complex systems and
their networks also expresses their diversified archetypal character
through the concepts of dynamical attractors and attractor landscapes.
These derive from analysis of data taken from observations and
measurments of actual systems that allow for creating abstract
mathematical models. Those models are represented as graphs which give
us another way of visualizing the characteristic behaviors of these
ultimately undefinable phenomena.
Network Dynamics as Dynamical Attractors
Consideration of network dynamics is
aided by the abstract notion of dynamical attractors. The activity of a
system over time can be measured and represented as a graph showing
cycles of movement. In simple physical systems, like a
swinging pendulum, the cycles appear in relation to a fixed point on
the graph. That point suggests a center around which system
activity "orbits," as if there were some central force controlling the
actions of the system--such as the swing of a pendulum. Thus it is
termed a dynamical attractor. But this location on a graph, around
which the activity of a system appears to be organized, is not a
literal force. It is an expression of the overall activity of the
system, of the dynamical relationships between its parts that are its
operational network.
A simple dynamic system with a fixed attractor point
A pendulum swinging in a circle, graphs of its initial activity around a
fixed attractor point at the center, then its changes as the dynamic force dissipates:
The graph of the pendulum
movement indicates its attractor point by tracking the activity of the
network of relationships between it parts as a system: the weight on a
string, the point from which it hangs, the velocity of its initial
impetus, and the centripital force of gravity resisting its momentum.
This image provides a means of vizualizing or seeing the system's
dynamical network, in contrast to the static network it manifests when
not in motion.
The swirling of water in a vortex provides another
example of dynamic network relationships. The moving water
interacts with the physical form of its container and the force of
gravity to manifest the network of relationships we see as the vortex.
Order formation in chaotic dynamics manifesting an attractor:
A vortex in water and diagram of the dynamic relationships of its
network formation around an attractor point:
The predictable patterns of activity in
systems having linear dynamics, like the pendulum, are said to manifest
a "steady state" that has a "fixed point dynamical attractor."
Systems whose activity repeats continuously are described as having a
"limit cycle" and a "periodic attractor." The dynamical behavior of the
system goes through a cycle then repeats itself. Both can be graphed in
two dimensions and express a constantly positioned attractor point on a
graph.
But when it comes to systems having more
non-linear dynamics and the disorderly ordering of chaos and
complexity, their pattern formation involves many more variables. It
becomes unpredictable. Representing the attractors of these systems
requires three-dimensional graphing in what is termed "phase space." In
effect, the attractor point of dynamic activity in such systems moves
around on the graph as the system's activity changes over time, yet
remains in a somewhat ordered pattern. There is no fixed point of
reference yet there is recognizable similarity in the successive
actions, or "phases," of the system's behavior. Yet the overall activity of the system
does generate an ordered form on the graph.
Systems expressing chaotic and complex
dynamics tend to generate graphs with multiple, sequential, and moving
attractor points. Their network of dynamic relationships is continually changing, yet remains within identifiable boundaries Such
variable attractors are termed "strange" due to the unpredictable yet
order creating behavior of the systems they help to describe. Systems
whose activity express strange dynamical attractors never exctly repeat
the same forms or patterns.
Simple and complex attractor patterns
Predictable fixed point and periodic attractor patterns versus
an unpredictable yet ordering strange attractor:
These mathematically derived images
provide ways of visualizing different types system behaviors that arise
from dynamical network relationships among system parts and external
factors
Dynamic patterns as response to "inner and outer forces"
Diagram of a repellor and attractor interaction, and a phase space map:
Understanding how
complex systems and their dynamic networks both fluctuate and yet
maintain
an identifiable overall form is aided by the concept of multiple and
sequential attractors. When a system is disturbed, or perturbed, its
operations can
change, generating a different attractor point. Then it might return to
the preceding pattern of activity, or reorganize again to yet another
one. This is referred to with the term "perturbation." What
is distinctive about complex adaptive systems is that their networks
can re-self-organize in response to perturbations. They often do so in
ways that either
restore the trajectory of a previous pattern or adapt to changing
conditions by generating a new network of relationships, which would
manifest as a different trajectory in a phase space map, with different
attractor points.
Here we can think of a
group of jazz musicians improvising around a melody. Each player is
concurrently and continually responding to the others while
occasionally improvising, from which emerges the on-going pattern of
their dynamic, self-determining yet unpredictable interplay. The
innovation of one perturbs the existing pattern, prompting a similar
yet different one as the ensemble of musicians responds to the
perturbation. The ability of complex systems to respond to
perturbations of their dynamic patterns while still retaining, or
regaining, identifiably similar forms is referred to as "robustness."
But such systems can respond to perturbation with abrupt metamorphic
change as well, "leaping" to a quite different pattern of behavior in a
sudden transformation.
System responses to perturbations
A perturbed system moving through an attractor sequence and
one making a transformative shift to very different mode of self-ordering:
This array of
abstract images helps reveal, by making "visible," the diverse archeytypal character
of dynamical systems and their networks. The graphs allow us to
visualize the ways their interdependent interactivity, among diverse,
even conflicted elements, produces order from disorder, resulting in
strange, variable, yet bounded forms and operations. But, of course,
these images are not the ultimately undefinable operations of the
networks that they model. In this regard, they are like the
symbols of mythic imagination.
Link to video animation of changing attractor landscape
Archetypal forms of dynamic behavior
Examples of the range of identifying symmetries of
pattern formation expressed as 3D graphs:
Network Dynamics In and As Dynamical Attractor Landscapes
A
further method
for visualizing the dynamical relationships of systems and their
operational networks extends to notion of attractors to include a
three-dimensional topography or landscape. This provides images of what
are termed
attractor basins and attractor landscapes. A literal example of an
attractor basin is a toilet
bowl in which moving water forms a vortex. But this concept also
describes the formation of dynamic system behaviors that are not
directly constrained by a physical container. The shape of a basin can
be an expression of a systems own internal relationships that result in
its form, such as water currents interacting, or of influences from
external factors, such as feedback from other systems in their local
environment.
Attractor expression as an external form
Ways of modeling an attractor basin on a 3D graph:
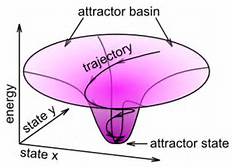
Attractor landscapes represent
complexes of attractors and repellors that are expressed by, or
exert influence upon, the ways a system network manifests its form and
operations. Perturbations of a system's existing pattern of dynamical
behavior can shift its network of dynamical relationships from one
attractor basin to another on an attractor landscape. But again, these
images do not necessarily represent specific physical forces. They are
a way of imagining the evolving interactions within and between systems.
Attractor proximity as a dynamic topology
Models of multiple attractor landscapes that influence system dynamics:
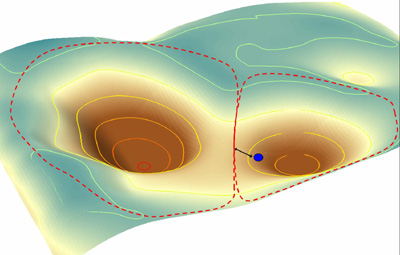
The complex dynamics in
some systems express multiple attractor points. When graphed, there are
several points around which the activities of the system appear to
orbit or be ordered. Recall the image is of a river having many
vortices and eddies, each taking on variable but similar forms
simultaneously, as in the images above. These concurrently interact,
influencing each other, and together constituting a meta-system and
network of order creating relationships. This image provides the
basis to think of a dynamical attractor landscape existing within or as
a continuous system and network as a terrain or topology of attractors.
The swirls in a turbulent river or numerous persons interacting
as a social group express a multiplicity of differing but interacint
pattern formations. These can be considered as constituting the
topological traits of an attractor landscape.
Conceiving how systems take form and operate
through these images, as the interactions of various dynamical
attractors, some of which are predictably constant and others
"strangely" unpredictable, elaborate our sense of network
operations. The on-going emergence of adaptive network autonomy
appears somewhat more logical when one thinks in terms how complex
attractors can "move about" and even "collaborate" within one system.
We can then conceive of many dynamical attractors interacting as an
attractor landscape manifesting from concurrent flows of feedback both
within a system/network and between it and the attractors of other
systems/networks.
Real World Attractor Landscapes
The notion of dynamical attractor landscapes is
useful in understanding the ways real world systems manifest their
network autonomy. A complex system like a court of justice is
constituted by many different sub-systems, each with their own specific
characteristics: law enforcement agencies, prosecutors, attorneys,
penal systems, judges, and a diversity of laws to enforce. Both these
components and the personalities of the individual persons involved in
them constitute a vast array of variably competitive and cooperative
interests. But they are also influenced by other systems that interact
with them: legislatures, politicians, social groups, news media,
cultural norms, etc. All these components and factors constitute a
complex, continually fluxuating attractor landscape. Depending upon
which dynamical attractors are activated at any given moment, the
overall meta-system can manifest very different traits of network
autonomy, of archetypal character and intentionality. And depending
upon the subject of a given legal case or the status of a defendant,
different dynamical attractors withing this landscape can be
activated. If a defendant is identified as a member of an ethnic
minority or lower ranked social class, the system is likely to behave
differently than if he or she is a member of an ethnic majority or
higher ranking social class.
The Illogical Relations of Humans to Complex Networks: Our Obsession with Control Equals Ignorance of Network Autonomy
Everything that makes us human, that constitutes the
archetypal character of the human body/mind system, is arguably a
property of complex network dynamics, rather than deriving only from
the physical matter and energy those dynamics organize into our
humanness. We are animated by our emergent networks. If we are to
know our selves, much less the world, if we are to think like Nature
acts, we require a form of knowledge about those dynamics.
Surprisingly, the scientific knowledge we have thus far gained about
them actually derives from our resistance to their implications. As
humans, we adapt to our environments by manipulating them. But we do so
not only through our physical abilities. We create technologies that
exponentially amplify our capacities for manipulation. We exist by
manipulating other natural systems, even each other, in an effort to
dominate and control them for our purposes. We are archetypally
"creatures of control," acting with out regard for how our behavior
impacts the network autonomies of other systems. What we deem is good
for us is the ultimate good.
As such creatures, science represents our quest for
ultimate knowledge about reality which will grant us ultimate control
over everything. However, that relentless pursuit of the knowledge of
control is what has generated the scientific evidence for the existence
of un-controllable network autonomy. Our analytical taking things apart
to understand exactly how they are constructed and happen has revealed
the very limits of our ability to fully understand, much less control
Nature. As logically accurate as this statement might be, it conflicts
with our cultural belief about how reality logically ought to be.
Despite the fact
that
scientific knowledge enables us to exponentially amplify our capacity
to manipulate and control many aspects of our environments, these
powers do not allow us to control the autonomy of complex networks.
Ironically, knowledge of science and technology is itself an emergent
property of
such networks, not simply a development or logically consistent
thought and calculation. Nonetheless, civilization is necessarily a
control-obsessed approach to the world. We tend to employ the
creativity of our own mental network autonomy to enhance our capacity
for control. Agriculture, industry, and
computational technology constitute emergent knowledge but as cultural
practices are based on direct manipulation of things and
events to maximize control. The success of such behavior leads us to
assume
all events can be and should be controlled. Thus we
continually attempt to create top-down, command and control systems. We
do it to each other as well as to natural systems. We create
competitive economies that drive the creation of ever larger
corporations seeking to dominate markets. The logic of
network science shows this is all a dangerous delusion. Sooner or
later, the autonomy of the system networks we thusly disable will react
disruptively or else collapse.
Our ignorance of complex network dynamics and
their essential autonomy is now revealed as a scientifically illogical
failure of our intelligence. It leads us to regard natural
systems as
machinery that has become organized only by the predictably
deterministic Laws of Physics and random chance. This view renders us
blind to the actual operations of complex networks and how our
manipulations disable these, resulting in ecological devastation, mass
extinction, and global climate change. Thus we desperately need a
compelling way to make ourselves more aware of network reality. That,
network science now shows us, is exactly what ancient cultures were
doing with the dynamical modeling of mythological symbolism and its
intuitive representation of network autonomy's archetypal
personalities.
|
|